OK-VQA
Outside Knowledge Visual Question Answering
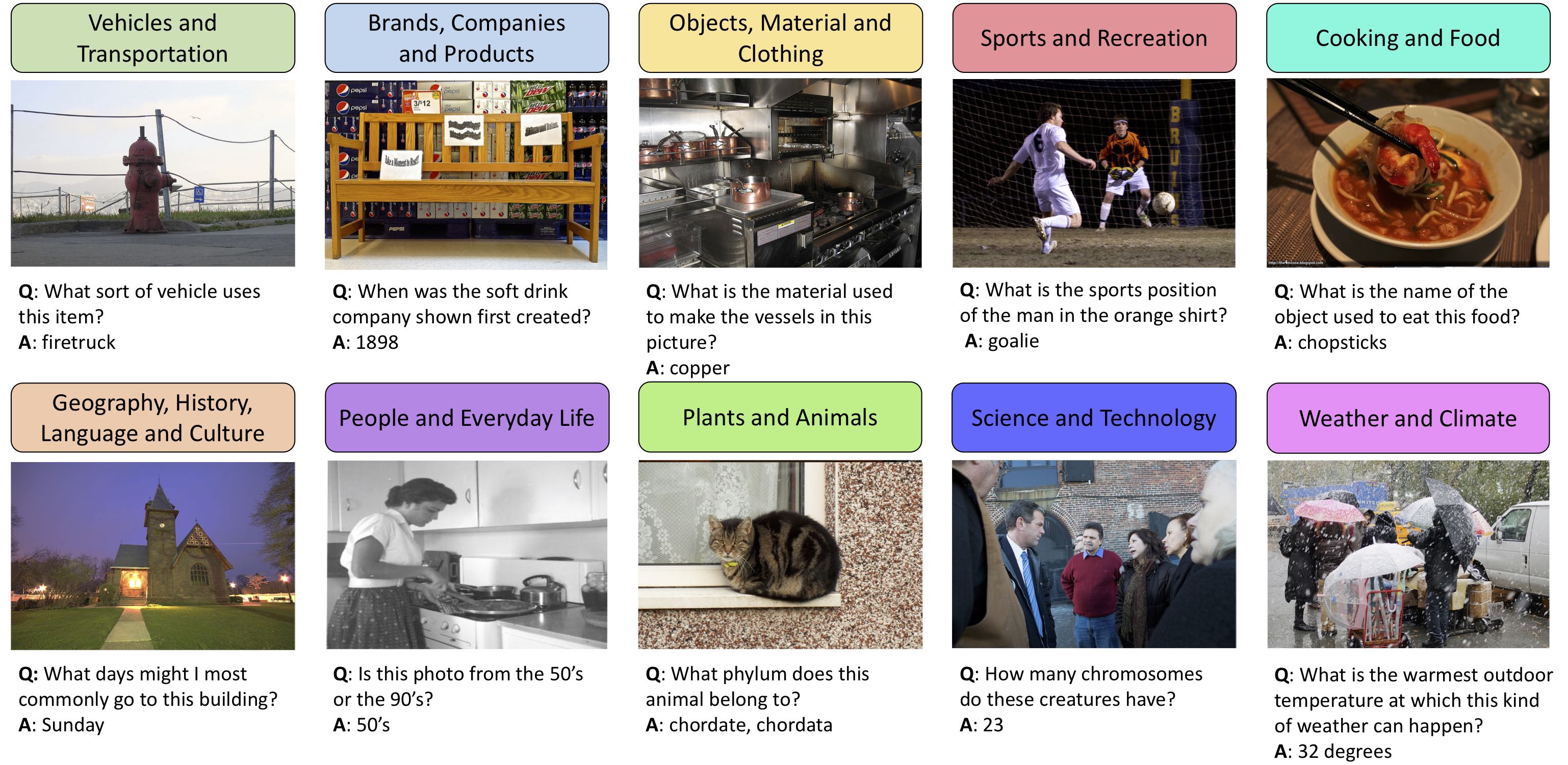
Summary
OK-VQA is a new dataset for visual question answering that requires methods which can draw upon outside knowledge to answer questions.
- 14,055 open-ended questions
- 5 ground truth answers per question
- Manually filtered to ensure all questions require outside knowledge (e.g. from Wikipeida)
- Reduced questions with most common answers to reduce dataset bias
Note: For A-OKVQA, the Augmented successor of OK-VQA, you should follow this link. A-OKVQA requires other types of knowledge and provides rationales for the answers in training. There are no common question-image pairs between A-OKVQA and OK-VQA.
Paper
OK-VQA: A Visual Question Answering Benchmark Requiring External Knowledge (CVPR 2019)
Visual Question Answering (VQA) in its ideal form lets us study reasoning in the joint space of vision and language and serves as a proxy for the AI task of scene understanding. However, most VQA benchmarks to date are focused on questions such as simple counting, visual attributes, and object detection that do not require reasoning or knowledge beyond what is in the image. In this paper, we address the task of knowledge-based visual question answering and provide a benchmark, called OK-VQA, where the image content is not sufficient to answer the questions, encouraging methods that rely on external knowledge resources. Our new dataset includes more than 14,000 questions that require external knowledge to answer. We show that the performance of the state-of-the-art VQA models degrades drastically in this new setting. Our analysis shows that our knowledge-based VQA task is diverse, difficult, and large compared to previous knowledge-based VQA datasets. We hope that this dataset enables researchers to open up new avenues for research in this domain.
Authors
Kenneth Marino, Mohammad Rastegari, Ali Farhadi, Roozbeh Mottaghi
Contact
If you have any problems or requests, contact kdmarino [at] cs [dot] cmu [dot] edu